Introduction
Artificial Intelligence (AI) is rapidly transforming industries worldwide, and the banking sector is no exception[1]. For mid-tier Australian banks, AI offers a unique opportunity to enhance efficiency, improve customer experience, and remain competitive against larger, well-funded institutions. This paper explores the current state of AI in the banking industry, identifies future opportunities for mid-tier banks, and discusses how these institutions can effectively integrate AI into their operating models.
Overview of AI
Artificial Intelligence refers to the simulation of human intelligence in machines designed to think and act like humans. These systems are capable of learning from experience, adapting to new inputs, and performing human-like tasks. In banking, AI encompasses a wide range of technologies, including machine learning, natural language processing, and robotic process automation (RPA).
The Current State of AI Deployment in the Banking Industry
AI is already being deployed across various functions within the banking industry. The key areas of application include automation, customer engagement, risk management, and customer proposition. Thus far, much of the AI deployment has been at large banks with deep pockets. Yet the mid-tier banks have an opportunity to move fast from here because of their relatively greater levels of agility and the availability of first rate technical and change management options.
Automation
AI-powered RPA bots automate repetitive, rule-based tasks such as document processing and data entry. These bots enhance accuracy, speed, and scalability, allowing human resources to focus on high-value activities. Current applications in the banking sector include customer on-boarding processes, account management, and loan processing. However, with the addition of AI capabilities to bots, a new generation of intelligent “agents” are emerging which combine AI and traditional RPA. This technology is known as Intelligent Automation (IA)[2]. IA provides enormous scope for process automation in banking.
Customer Engagement
Banks have moved from basic AI applications to more complex machine learning and deep learning systems[3]. These technologies enable predictive analytics, helping banks make informed strategic decisions based on real-time data. AI also enhances customer experience by providing personalised services and support around the clock. Emerging chatbots are an order of magnitude better than the clumsy older technologies. However, there does need to be a material effort invested in training.
Risk Management
AI plays a critical role in risk management by improving the accuracy and speed of credit risk assessments, market forecasting, and fraud detection[4]. Machine learning algorithms continuously learn from new data, outperforming traditional methods in identifying fraudulent transactions and cybersecurity threats. This creates an opportunity for “real time compliance” at considerably lower cost. The “gotcha” here is that it helps enormously to be on top of your processes to be able to deploy effectively.
Examples and Use Cases
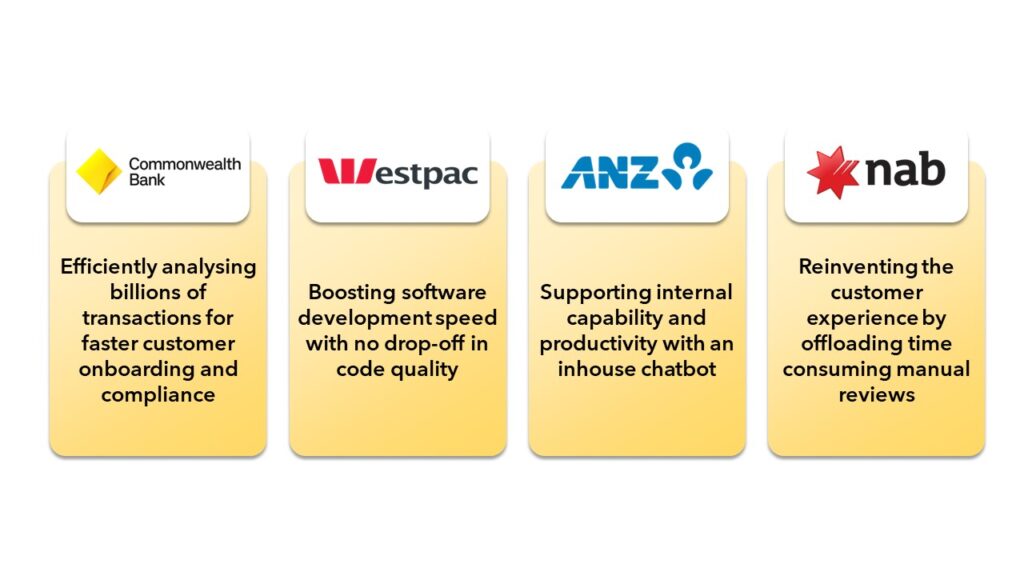
Figure 1: The big 4 banks are deploying AI solutions across a variety of applications.
Several banks have successfully implemented AI solutions. For instance, the Commonwealth Bank of Australia uses H2O.ai’s Document AI to analyse transactions and process documents efficiently[5]. Westpac has leveraged generative AI tools to enhance productivity and introduced AI-based questionnaires to combat fraud[6]. ANZ’s in-house chatbot, Z-GPT, boosts efficiency in various tasks[7], while National Australia Bank uses generative AI for customer complaints assessment and personalised messaging[8]. NAB are also using AI to generate proposals for business customers.
Challenges
Despite its benefits, AI adoption in banking faces considerable challenges. Some common themes are technical challenges (integration with existing systems, model interpretability, model scalability, and performance reliability are significant hurdles), ethical challenges (data privacy, AI biases, and regulatory compliance issues need careful consideration), and AI-aversion challenges (there is a general mistrust of AI among consumers and regulators, which can hinder its acceptance and implementation. This may be particularly pronounced in Australia [9].)
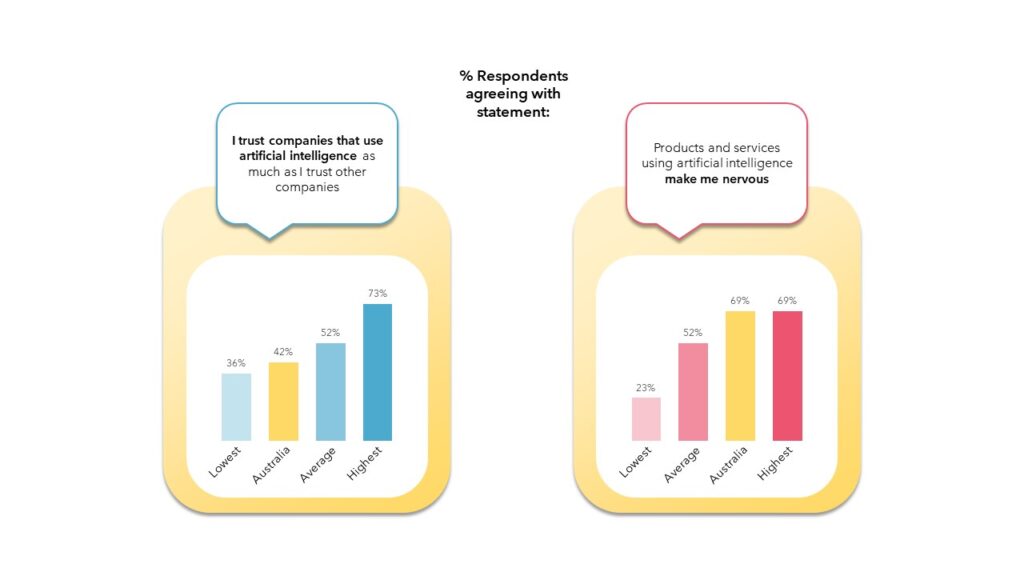
Figure 2: In a recent survey covering 31 countries, Australian respondents were some of the most AI-averse in the world.
Future Opportunities for Mid-Tier Banks
While larger banks have the capital to place larger AI related bets, mid-tier banks have the potential to leverage AI to punch above their weight by focusing on their nimbleness compared to larger competitors. The key opportunities for AI deployment include strategic partnerships, high-value AI opportunities, and fostering flexible and agile innovation.
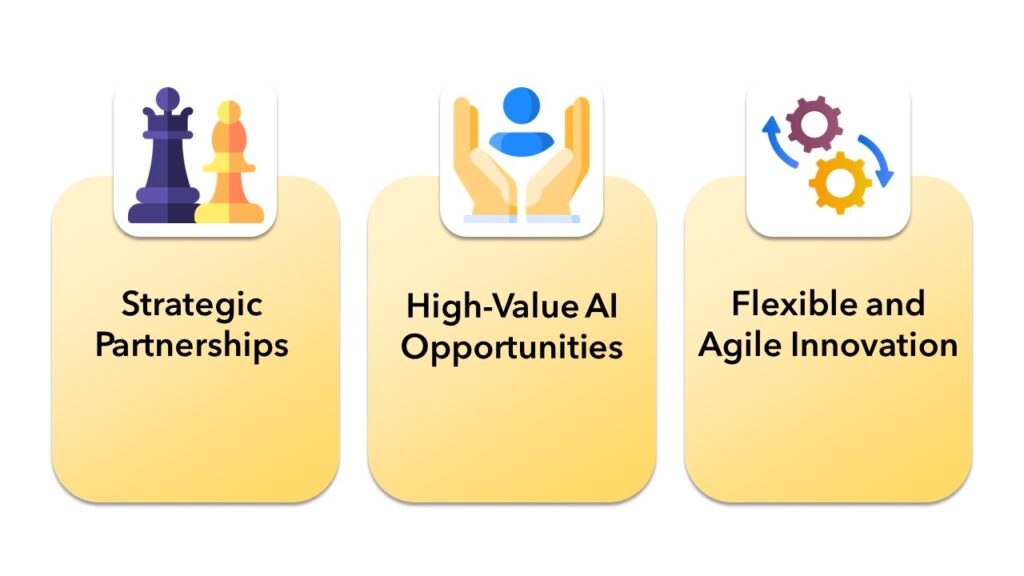
Figure 3: Mid-tier banks should focus on opportunities aligned to their increased agility, relative to larger institutions.
Strategic Partnerships
Collaborating with technology providers and AI specialists can help mid-tier banks overcome technical challenges and accelerate AI integration. These partnerships should focus on finding tailored solutions that go beyond basic process automation to address specific customer problems. Often it helps to have a specialist work with you on making the right choices here. A wrong choice can cost time, and this space is particularly time sensitive. Those organisations that can get to market early can seize the higher ground in the customers’ minds.
High-Value AI Opportunities
Mid-tier banks can explore high-value AI opportunities in areas such as fraud detection, credit risk assessment, personalised customer experience, and process automation. Investment in AI can significantly reduce operational costs, enhance productivity, and offer hyper-personalised services to customers. Once again good early decisions matter here. The best approach is to develop a portfolio of projects with different target benefits and degrees of difficulty. Naturally, the higher benefit opportunities with lower implementation difficulty should be prioritised. This does presume some technical and commercial knowledge with regards to assessing degree of difficulty.
Flexible and Agile Innovation
Due to their smaller size, mid-tier banks can rapidly adapt to regulatory changes, tailor AI-driven products to specific customer needs, and differentiate themselves from larger competitors. This flexibility allows them to implement AI solutions quickly and effectively, providing a competitive edge in the market. The trick here is to ensure the organisational structure and ways of working facilitate fast and effective innovation and commercialisation. It seems that, in practice, agile ways of working have real speed and flexibility benefits.
AI and the Operating Model
An operating model is so much more than structure. It also incorporates capabilities, technologies, ways of working, and culture. To fully harness AI’s potential, mid-tier banks must integrate it into their operating models. This requires a comprehensive approach that considers workforce implications, technology infrastructure, data management, and regulatory compliance. Specialist operating model advice is recommended here as this is complex subject matter and most leaders are rarely called upon to engage deeply in this subject (so there has been little recent practice or understanding of the contemporary options).
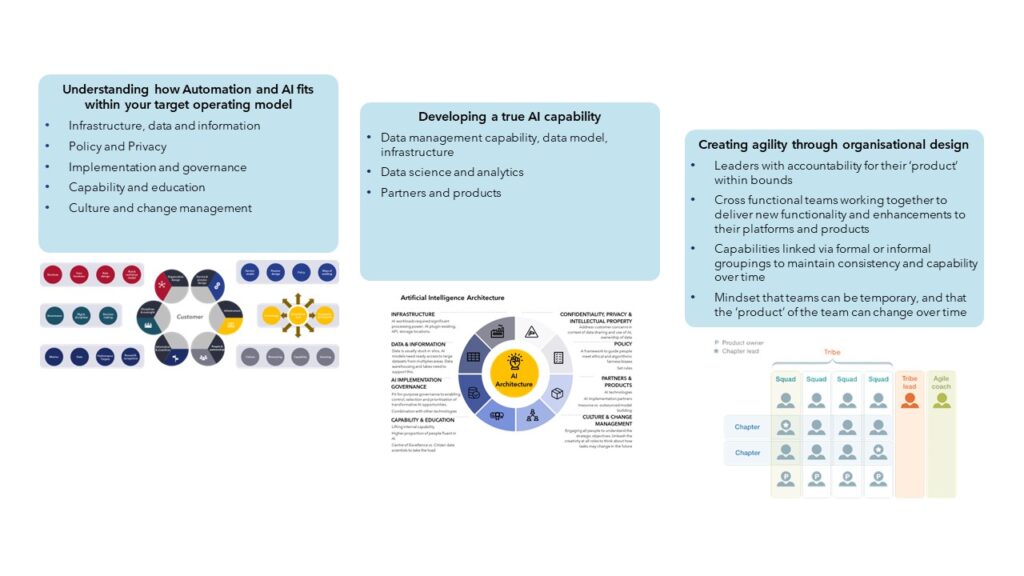
Figure 4: AI implementation can benefit greatly from an operating model first approach.
Impact on Operating Model
AI affects all elements of the operating model, including organisation design, service and process design, infrastructure, people and partnerships, information and incentives, and disciplines and oversight. A well-designed operating model will enable banks to deploy AI more rapidly and effectively.
Workforce Changes
The implementation of AI will result in significant changes to the workforce. Roles requiring routine tasks may be automated, while those demanding higher cognitive skills will become more prominent. Training, upskilling, and change management are crucial to ensure a smooth transition. It is increasingly common for organisations to now update their workforce plans with AI very much in mind. A workforce plan should outline the shape of the future workforce (roles, numbers of personnel, skills, etc.) and how the enterprise plans to get there.
Regulatory Compliance
Adhering to regulatory standards is essential for successful AI integration. Banks must ensure that their AI systems comply with relevant regulations and prioritise data security and ethical conduct. Transparent communication with customers and regulators will build trust and confidence in AI solutions. Note that laws and regulations will be emergent in this space. To get a sense of where Australia might go it is worthwhile familiarising yourself with the rules recently issued by the European Union[10][11].
Conclusion
AI presents a significant opportunity for mid-tier Australian banks to enhance their operations, improve customer experience, and remain competitive. By leveraging their nimbleness, forming strategic partnerships, and investing in high-value AI opportunities, these banks can effectively integrate AI into their operating models. Overcoming technical, ethical, and regulatory challenges will be crucial to realising AI’s full potential. With a well-designed operating model and a focus on workforce readiness, mid-tier banks can harness AI to achieve significant operational efficiencies and deliver superior value to their customers.
References
- Roger Perry. How to Respond to AI changing your operating model. 2023. url:https://bevingtongroup.com/article/navigating-the-transformative-ai-wave/.
- IBM. What is intelligent automation? 2023. url: https://www.ibm.com/topics/intelligent-automation#:~:text=IBM-,What%20is%20intelligent%20automation%3F,scale%20decision%2Dmaking%20across%20organizations.
- Australian Fintech. Chatbots just the beginning for AI in banking. 2018. url:https://australianfintech.com.au/chatbots-ai-banking/.
- Max Liul. AI in Banking Risk Management: Popular Use Cases. 2022. url: https://integrio.net/blog/ai-in-banking-risk-management.
- Liz Pratusevich. How Commonwealth Bank is transforming operations with Document AI. 2023. url:https://h2o.ai/blog/2023/how-commonwealth-bank-is-transforming-operations-with-document-ai/.
- Kate Weber. Westpac sees 46 percent productivity gain from AI coding experiment 2023. url:https://www.itnews.com.au/news/westpac-sees-46-percent-productivity-gain-from-ai-coding-experiment-596423.
- Tim Hogarth. Adapting the artificial intelligence model. 2023. url: https://bluenotes.anz.com/posts/2023/08/anz- news- tim-hogarth-zgpt-chatbot-australia.
- Anna Cuisia Antoine Amend Robin Sutara. Simplifying Data Governance in AI-driven Financial Services. 2024. url: https://www.databricks.com/blog/simplifying-data-governance-ai-driven-financial-services.
- Ipsos. Global vies on A.I. in 2023. 2023. url: https://www.ipsos.com/sites/default/files/ct/news/documents/2023-07/Ipsos%20Global%20AI%202023%20Report%20-%20NZ%20Release%2019.07.2023.pdf.
- European Commission. Shaping Europe’s Digital Future. 2023. url: https://digital-strategy.ec.europa.eu/en/policies/regulatory-framework-ai.
- European Parliament. EU AI Act: first regulation on artificial intelligence. 2023. url: https://www.europarl.europa.eu/topics/en/article/20230601STO93804/eu-ai-act-first-regulation-on-artificial-intelligence.
![]() |
Roger Perry – CEO Bevington Group One of the region’s foremost productivity improvement and organisational design experts, focused on ensuring successful organisational change, with demonstrated and quantified returns. |